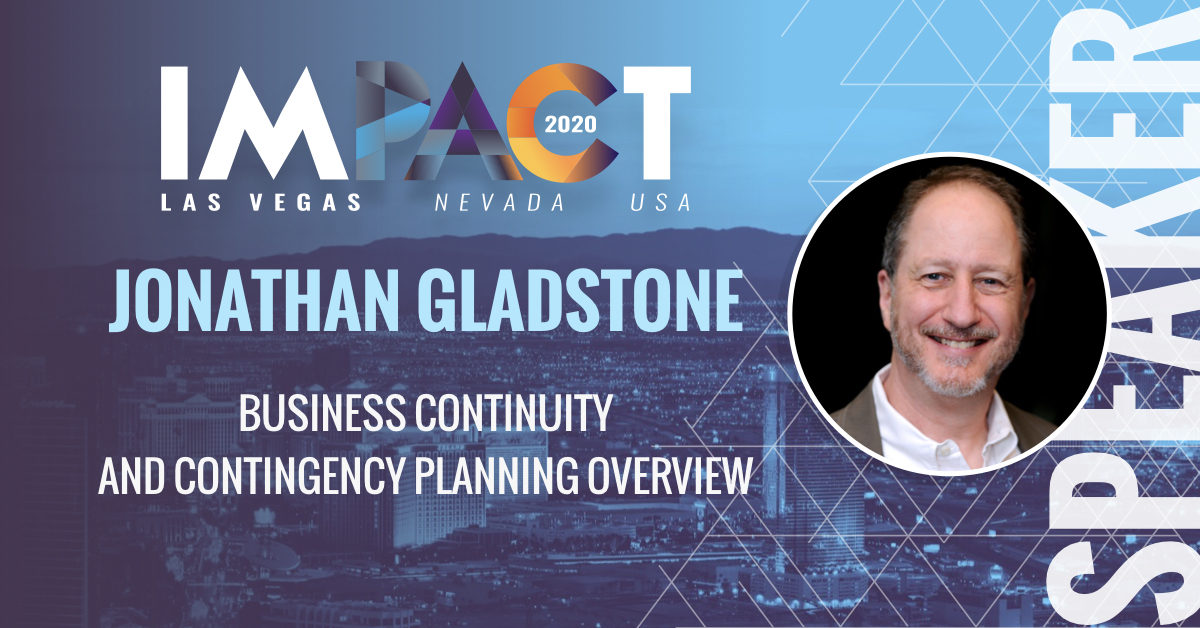
IMPACT2020 | Business Continuity & Contingency Planning or, Who Turned out the Lights -Jonathan Gladstone
September 27, 2022
How to prepare your business for the Metaverse and for Web 3.0
September 27, 2022Geometrical projections of the transformations of the data space during the execution of the families of algorithms represented by Isolation Forest, Random Forest, and Neural Networks are explained to expose the hidden weaknesses of the conventional approach using hyperplanes as decision boundary.
In this session, the family of algorithms represented by Isolation Forest is presented as a case study to highlight the hidden weaknesses that would manifest in the false identification of outliers in numerical data, such as performance metrics.
Synthetic data is used to amplify the hidden weaknesses of the conventional use of hyperplanes as decision boundaries and the improvements by the proposed modifications of using geometrically appropriate decision boundaries are confirmed by comparing the results from applying the algorithms to an online credit card fraud dataset.
Using only 7 features of the credit card fraud dataset with properties suitable for the metric, the modified algorithm outperforms the conventional algorithms using hyperplanes as decision boundaries with all the 28 features as input, demonstrating the strength of the proposed modifications.
Presented by
IMPACT 2023 Proceeding Session Video:
To view the proceeding session video you must have a CMG Membership. Sign up today!
For existing members sign in here.